Manual Therapy Online - Articles
Methods of Clinical Reasoning
The following are the most used methods to reason through a patient’s problems with each being addressed to different extents in the following chapters:
- Algorithms including:
- Flow charts
- Treatment based classification schemes
- Protocols
- Triaging methods
- Heuristics
- Pathognomonics
- Pattern Recognition
- Hypothetic-deduction
A sixth method, Script Focused Deduction (SFD) will be introduced in this book.
Each of these methods has its place according to the expertise and experience of the clinician, the patient and his/her problem, which signs and symptoms occur first in the examination. No one method will work for every clinician or every problem at all times. For example pattern recognition can only occur when the problem that is encountered has been seen in sufficient numbers by the clinician for her/him to recognize it without moving into hypothetic-deductive reasoning; for this reason pattern recognition is mainly confined to experts. Pathognomonics is a method of opportunity, the particular sign/symptom has to be seen for it to be used.
In addition to the methods clinical reasoning another factor must be considered, cognitive error caused by insufficient or inaccurate knowledge and bias, an unconscious lean towards a particular result. In the literature there are some forty bias’ documented but this book will only discuss the most relevant to the subject at hand:
- Availability
- Anchoring
- Search satisfaction or premature closure
- Framing
- Base rate neglect
These need to be recognized and managed by the clinician which is difficult given that they are unconscious and ingrained but hopefully this book will deal with them by making their correction an integral part of the reasoning process in diagnosis.
The next chapter will get through the methods of clinical reasoning that really have nothing to do with reasoning, the algorithms and protocols. These methods simply give direction that is followed by the clinician. As such they are really more appropriate for the technician rather than the thoughtful clinician.
Clinical Non-Reasoning: Algorithms, non-pathological classification schemes and such.
We will not spend much time on this subject as it has very little to do with clinical reason. These devices have, for the most part, their purpose in reducing errors made by students and novices in problem solving, increasing efficiency and cost effectiveness, standardization and sometimes simply because the teacher is unable to clinically reason let alone teach it. As with all methods algorithms have their strengths and weakness’ but in my view the weakness’ far outweigh their strengths, the main weakness being the student never learns to problem solve when the algorithm fails as it inevitably must.
Algorithms
These are essentially step-by-step instructions on solving a problem. Initially used in mathematics and later in computer sciences they are now popular in any number of non-mathematical tasks where an end point is to be achieved by a series of discrete operations. An extremely simple example is seen below, fixing a faulty lamp.
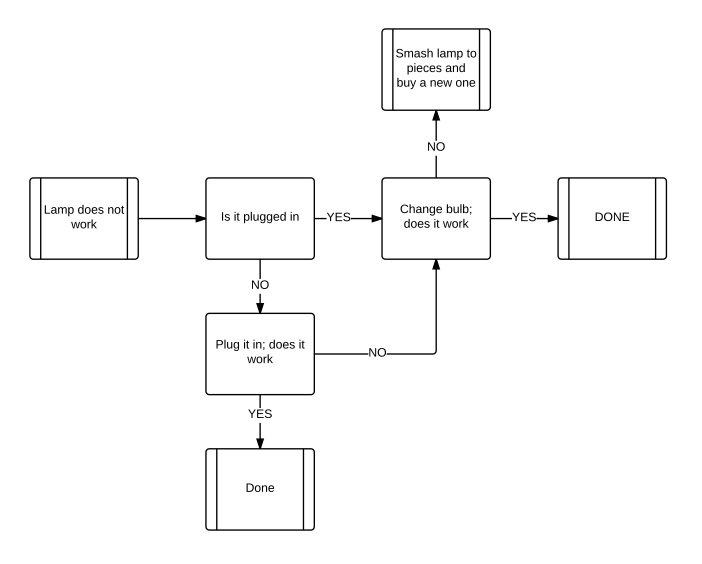
This is an algorithm in flow chart form and is used to visualize a process in the hopes of making the underlying use of the algorithm is clear, follow each step and you will come to a resolution of the problem but only as far as the flow chart goes. You can see how this would be useful for somebody who has little or no experience with lamps that do not work by working through the algorithm with each change asking the same question, “does it work” the novice will either make the lamp work or replace it with a working lamp. In either case there will be light. But what if the lamp is too expensive to be discarded or what if the problem lies with the wiring to the lamp, or the plug is faulty then a lamp will have been replaced that was not faulty. But light will be restored at the cost of a lamp.
Below is a more pertinent algorithm for assessing a posttraumatic headache for serious injury (intracranial bleed, traumatic hydrocephalus, vertebrobasilar ischemia).
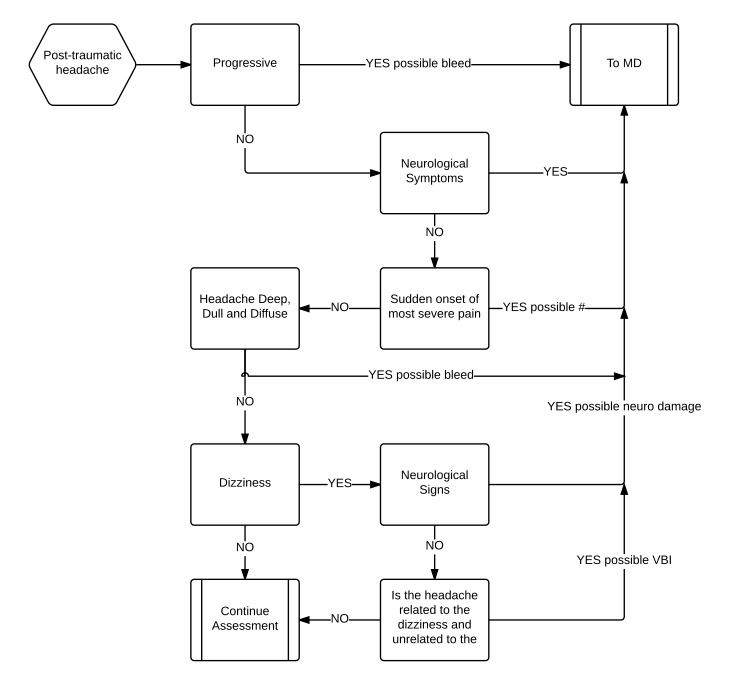
Our patients are not replaceable and if the patient’s problem falls outside of the scope of the algorithm then it will not be fixed. Even this might not be to much of a problem providing the clinician is not dependent on the algorithm and can think outside of it so that when it fails he/she can solve the problem by reasoning. But now the question becomes if he/she could do that what was the purpose of the algorithm. Well algorithms have other strengths beside being easy to follow, they eliminate bias and other cognitive errors from the equation. Cognitive errors are unconscious mistakes based on faulty reasoning, incorrect or inappropriate information while bias’s are preset mental and emotional conditions that might be useful (sensitizations) but in this context lead to errors. These will be discussed in more detail later. By following the algorithm the clinician has no chance of error or bias entering the picture providing the algorithm’s designer was free of error. So we can see that the strength of this type of algorithm is that it is visual and so makes recognizing the process more natural, it is relatively simple in that it keeps the actions within strictly defined parameters and it is free of bias but what of weakness’. Well the first and most obvious is that the problem has to be relatively simple or the algorithm becomes so complex that it would be easier to use reason to solve the problem. This means that when the algorithm fails the clinician must be able to work through the problem in a more traditional manner or must ask for help. The problem with this is that if the clinician has come to depend on the algorithmic approach to problem solving when will he/she ever learn to do anything else. That is using algorithms in this way dumbs down the practitioner and this might be its greatest weakness. In medicine where algorithms are often used particular during the training of physicians the literature on the subject places great emphasis on ensuring that the student knows that they must be able to step outside of the algorithm when it is not working for them. It then becomes an aide that is gradually discarded with more experience.
Below is an example of a flow chart algorithm for the treatment of a lumbar segmental dysfunction by manipulation.
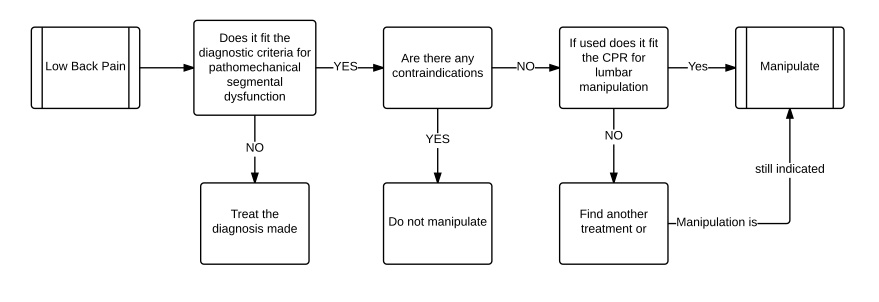
At present I am avoiding a discussion of the clinical prediction rule for lumbar manipulation but I have included it here for completeness sake. As you can see this algorithm is as clear as the previous but each step is quite complex; what are the diagnostic criteria, what are the contraindications and what is the prediction rule. These are not spelled out and the flow chart assumes that the follower of the algorithm will be familiar with them or failing that have an algorithm for each of them. But the “if this, then that” approach of the algorithm is present in a flow chart form.
Decision trees are another form of algorithmic flow chart, which outside of mathematics is probably most useful in determining the effects of actions, so it is mostly used for planning operations rather than carrying them out.
Algorithms can be expressed in other than flow charts and we have two obvious examples of that in physical therapy. The first that most orthopedic therapists are familiar with is the clinical prediction rule in lumbar manipulation. Effectively this states that providing certain criteria are met the chances of manipulation providing pain relief is at a given level. The fewer criteria met the lower the chances. In effect this algorithm is simply a list of data that must be collected by the clinician from the patient. The second is the classification of low back pain according to what was found in the subjective, objective and imaging/lab examinations, with these classifications requiring a set treatment. This method effectively does away with a pathoanatomical diagnosis in favor of a syndrome approach to the management of low back pain patients. It goes a step further then the clinical rule that is simply prognostic in that the clinician must still collect the data from the patient and then see which grouping category affords the best fit for treatment and then carry out the predetermined treatment. This can be converted to flow chart form so:
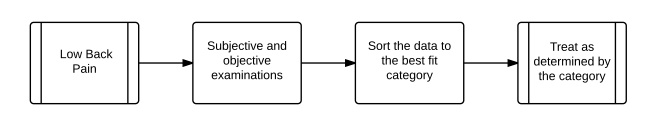
Both systems, the algorithm and pathoanatomical diagnosis have serious weaknesses with former being, in my opinion at least, decidedly counterproductive and harmful. The clinical prediction rule in its current incarnation suffers mainly from its lack of value to the clinician. The rule simply says that once that decision is made the chances of success is such and such depending on how many of its variables are present. It is not a diagnostic tool (although I have recently heard one of it’s chief architects mistakenly use it as such) and so the decision to manipulate must be made by diagnosis prior to using the prediction rule. Assume for the sake of this argument that there are none of the criteria present what do I do? I can either manipulate or not but if the diagnosis requires manipulation what are my options, mobilization, exercises or something else. We have to assume that based on our models and current best evidence the preferred treatment is manipulation and in the absence of contraindications this is still the treatment of choice. If this ever becomes part of a larger risk-benefit assessment tool then it might be helpful but at present it is a waste of time; clinically anyway, as a political tool it had and has immense value.
But at least it is fairly harmless which is more than can be said for the classification method. This has so many failings that it is difficult to know where to start but I will concentrate on the two most glaring. First most patients that we see are more complex than a simple this or that condition. There are often two or more conditions that might be independent or interdependent, there are often hidden etiologies that need to be identified and managed, there are personality issues that affect the interaction of the therapist and patient, there is the past experience of the patient with a given treatment that causes she/him to not want the determined treatment. In short people do not fit into neat pigeonholes and neither do their problems. So trying to sort out a complex case by using this method is more complicated than trying to reason your way through it using non-algorithmic processes. The second major issue is that if the student becomes dependent on this method and is not using clinical reasoning in the simplest cases where this method might serve reasonably well, when are they going to develop the ability to work things out and problem solve. Dumbing down a complex problem is patronizing at best and an indication of the teacher’s incompetence’s at worse; in either case it is unpardonable.
However, algorithms really do have their place. An example of this is Goldman’s algorithm. This is a mathematical heuristic for the triage of acute onset chest pain in the emergency room. Goldman went into the literature and researched hundreds of cases of acute chest pain to find the key questions and tests that would lead to safe and cost effective treatment of the serious patient who was potentially at risk of imminent death. He found the following to be extremely sensitive and very specific for acute myocardial infarctions; unstable angina, fluid in the lungs and a systolic blood pressure below 100. The algorithm was used in Chicago’s Cook County hospital with great success outperforming the physician’s personal approach considerably, a finding that has been upheld on at least one occasion. (Qamar, A. McPherson, C. et al. The Goldman algorithm revisited: prospective evaluation of a computer-derived algorithm versus unaided physician judgment in suspected acute myocardial infarction. Am Heart J. 1999 Oct;138(4 Pt 1):705-9.
Goldman completely ignored predisposing features such as weight, obesity, family history etc and concentrated on the minimum criteria that would diagnose the condition. He weighted each criterion according to a mathematical heuristic and made recommendations on the management of chest pain patients according to which category they fell into. This is the same system as previously discussed but it is worth mentioning that the method was formulated on extensive research of the literature and a rigorous mathematical treatment and was instituted by qualified physicians rather than taught to students. In addition and probably more relevant this is a triage algorithm of the same type as the Ottawa knee and ankle rules, the NEXUS LRC and the Canadian C Spine rules, rather than a diagnosis and treatment algorithm.
This study is a major factor in the development of the script focused deduction method that we shall meet later. The idea that less information can be more useful than more information is really quite novel and controversial but here it seems to work.
Worth mentioning is the use of algorithms for training and learning purposes, not as set pathways to assess and treat patients but rather as a method of understanding relationships between signs and symptoms and processes. This can go in any direction, the teacher can draw up a flow chart of a particular process and then explain how these processes work together to integrate the data collected. The flow chart can be drawn up as a partnership between the teacher and the students and the students can draw one up to cement their understanding. I use this method to reduce processes down to the simplest visual that still contains all the variables present so that I know I fully (or at least as fully as I know anything from one moment to another) understand the process, at least on an operational level.
To summarize set algorithms have their place in diagnosis and treatment. They have strengths, notably they permit the visualization of relationships, processes and consequences and are excellent teaching and learning aids, they are simple and they are bias and error free when designed and used correctly. Their weakness’ include their non-comprehensiveness, that is they can really only deal with relatively simple problems (the Goldman algorithm for example simply sorts patients into one of three treatment groups), they tend to cause dependency and when used by a student often become the only tool they have with no opportunity to develop any others. Perhaps their worse sin is that using them is completely boring and when they work really well require technicians not innovators and thinkers who enjoy problem solving.
comments powered by DisqusFeatured Course
FREE COURSE
Foundations of Expertise
Date:
Starting March 24, 2024
Location: Online
LEARN MORE